State Space Surrogate model for Uncertainty Quantification of Stochastic Dynamical Systems
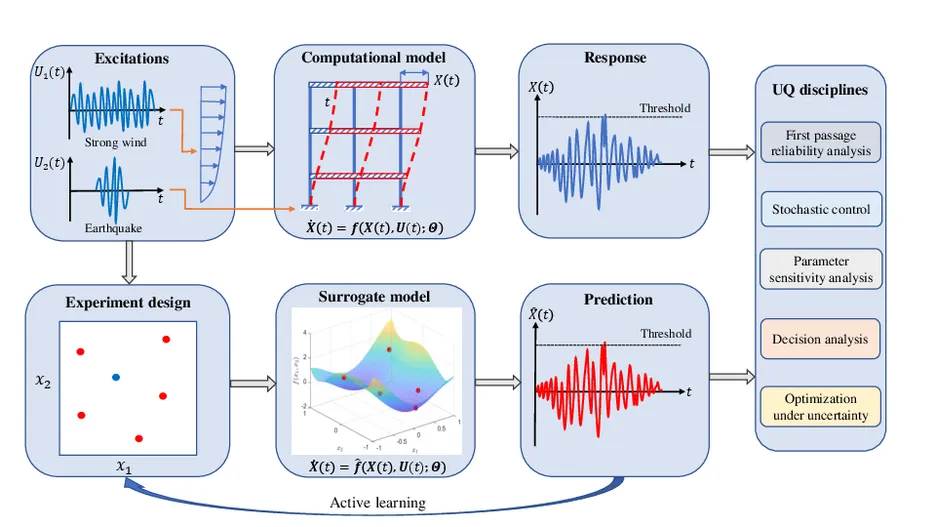
Engineering structural systems are subjected to various extreme loads during their lifetime, such as earthquake and typhoons loads on building, tsunami loads on dam, and on so. System failures due to these loads usually cause large-scale loss of life and property. Understanding of how systems fail and how likely they fail are therefore of paramount importance to prevent the loss. Although numerical simulation offers a feasible way to predict and study the performance of complex structural systems, their performances are governed by various intrinsic uncertainties associated with the external loads and the model parameters, e.g., stiffness and damping.
Uncertainty quantification (UQ) offers a comprehensive framework for studying the impact of uncertainties on system response quantities of interest (e.g., displacement and velocity). UQ requires repeated evaluations of the costly computational model, which is infeasible in practice. To address this issue, surrogate models are widely used to construct computationally efficient approximations of the expensive computational model, and propagate uncertainties efficiently. However, existing surrogate models are inefficient for high-dimensional problems, a challenge known as “curse of dimensionality”. For stochastic dynamical systems, the number of input parameters resulting from the discretization of stochastic excitations can be extremely high. This complexity hinders the application of existing surrogate modeling techniques to these problems. Therefore, development of efficient surrogate modelling techniques for UQ in complex nonlinear stochastic dynamical systems over long time period of interest, is a global open scientific challenge.
The current project: State Space Surrogate Model for Uncertainty Quantification of Stochastic Dynamical Systems (S3UQDyn), aims at overcoming the “curse of dimensionality” by developing an innovative surrogate modeling framework to emulate the response of complex nonlinear stochastic dynamical systems under uncertainty. This framework will enable engineers to establish digital twin models to monitor, control and maintain various dynamically excited critical infrastructures in real time during their lifetime, so as to avoid catastrophic event and reduce societal risks.
Researchers
Funding
Alexander von Humboldt Post-Doctoral Fellowship (AvH Foundation)
Publication
Kai Cheng, Iason Papaioannou, MengZe Lyu, Daniel Straub. " State Space Kriging model for emulating complex nonlinear dynamical systems under stochastic excitation, arXiv preprint arXiv:2409.02462 (2024).