Probabilistic Ground Model for Offshore Wind Farms
The development of offshore wind farms demands a comprehensive understanding of subsurface conditions, typically achieved through extensive geological, geophysical, and geotechnical surveys. These traditional approaches are time-consuming and costly, often requiring repeated assessments as turbine locations shift. To streamline this process and support the rapid deployment of offshore wind energy in Germany, this project aims to develop a probabilistic ground modeling framework that integrates diverse site-specific datasets and generic soil databases to infer subsurface conditions, including stratigraphy and soil mechanical properties, across entire wind farm areas.
The proposed framework combines advanced statistical and machine learning models, such as multivariate Gaussian Processes and numerical algorithms to balance computational efficiency with accuracy and uncertainty quantification, particularly for 3D subsurface volumes and multivariate datasets. Emphasis is placed on characterizing subsurface stratigraphy and seabed discontinuities, such as boulders, which are critical for monopile design in complex ground conditions. The model incorporates data from geotechnical, geological, and geophysical surveys alongside engineering knowledge to quantify and propagate uncertainties, supporting robust geotechnical assessments. This study aligns with the shift towards data-centric geotechnics, leveraging modern data science and machine learning techniques to enhance offshore wind farm design and risk assessment.
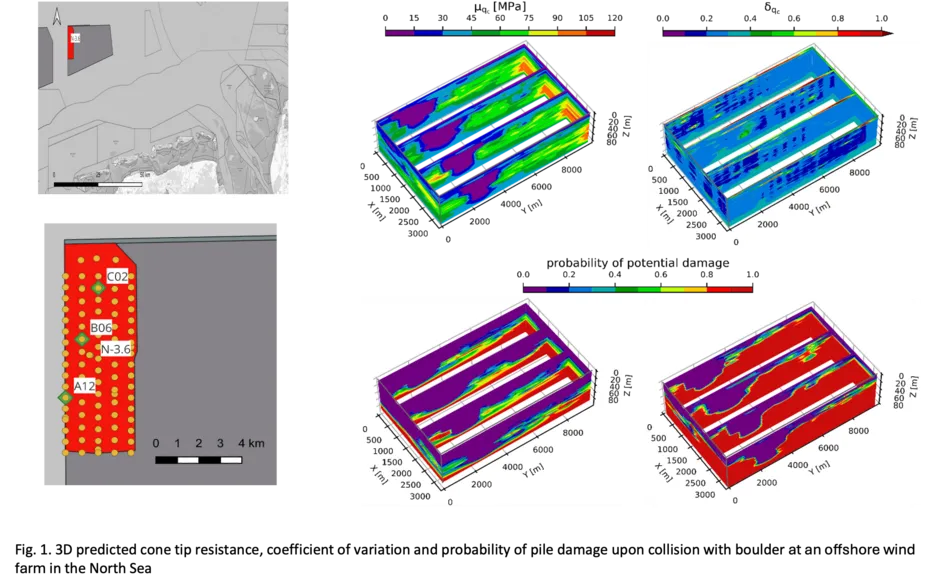
Researchers
- Orestis Zinas (BAM)
- Iason Papaioannou
- Ronald Schneider (BAM)
- Pablo Cuéllar (LfU)
Funding
This project is funded by the Bundesanstalt für Materialforschung und -prüfung (BAM).
Publications
Zinas, O., Papaioannou, I., Schneider, R., Cuéllar, P., 2024. Multivariate gaussian process for 3d subsurface stratigraphy prediction from cpt and labelled borehole data, in: 7th International Conference on Geotechnical and Geophysical Site Characterization. URL: https://www.scipedia.com/public/Zinas*_et_al_2024a
Zinas, O., Papaioannou, I., Schneider, R. and Cuéllar, P., “Multivariate Gaussian Process Regression for 3d Site Characterization from CPT and Categorical Borehole Data”. Available at SSRN: https://ssrn.com/abstract=4995360